5 Reasons Why Enterprise Generative AI Projects Fail
Sector: Data Analytics and Artificial Intelligence
Author: Nisarg Mehta
Date Published: 08/06/2024
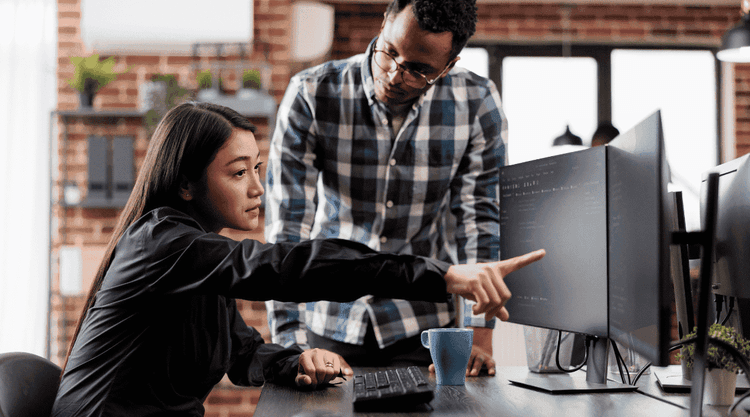
Contents
Every enterprise today wants to get on the road of AI-enabled operations or some form of automation. A recent study by IBM shows that 42% of large-scale organizations have already implemented AI capabilities while an additional 40% are quite active in considering AI implementation. These factors have made AI highly relevant and 59% of the companies have reported increasing their AI investment and deployment.
However, this enthusiasm for AI is often met with limitations. One of the finest real-life examples is LEVIDOW, LEVIDOW & OBERMAN, P.C., a law firm in the US that experienced dire consequences as the lawyers there provided legal opinions that contained fake citations and quotes sourced through ChatGPT. The firm and its lawyers were also ordered to pay $5,000 for rejecting their duty and relying on bogus judicial precedents.
One typical problem observed in enterprise generative AI projects is the difference between the set objectives and the achieved results. This blog will intend to look at the main causes of failure for such projects and some of the things that one should avoid to prevent the projects from failing.
So without further ado, let’s get started.
Reasons Why Enterprise Generative AI Projects Fail and Their Solutions
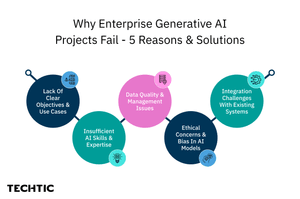
Let’s explore the key reasons.
1. Lack of Clear Objectives and Use Cases
Lack of purpose and direction is one of the significant causes of generative AI project failure. A lot of organizations jump into AI without knowing what they want to get out of it. This leads to situations where projects are not aligned with the business objectives and do not serve the intended purpose.
Lack of adequate AI skills and knowledge is cited as a major challenge by 33% of firms, demonstrating the importance of having a clear plan on how to use AI appropriately.
For instance, an enterprise may adopt AI just because the technology is popular in the market without the need to find a proper use for it. This lack of direction can lead to the project getting off track and ultimately failing.
How to Avoid This:
All AI initiatives should have clear, measurable goals set before the project begins. Create a well-coordinated and realistic approach that supports your organizational objectives. This helps make sure everyone knows why the project is being done, and what will be considered as success.
2. Insufficient AI Skills and Expertise
However, one of the largest challenges to successful enterprise generative AI projects has been that there are not enough AI talents and knowledge. Nearly a third of firms claim that a lack of sufficient AI expertise hinders them from realizing the full potential of deploying AI. It is often difficult to design, deploy, and sustain AI systems because of the lack of the right type of knowledge.
How to Avoid This:
- Invest in Training: Continually invest in training and development initiatives to source much-needed AI expertise internally.
- Hire Experts: Outsource some tasks or seek help from third-party AI development companies or consultants if necessary.
- Foster a Learning Culture: It is essential to foster a culture of learning and creativity in the organization.
3. Data Quality and Management Issues
Accuracy and handling of data in generative AI solutions define its efficiency and effectiveness. One of the main problems affecting many enterprises’ generative AI initiatives is data complexity which has been cited as a major challenge by 25% of respondents. Lack of data quality and failure to adhere to proper data management procedures compromise the result of the AI algorithm and decision-making outcomes.
For example, if the training datasets used in developing an AI model are either prejudiced or contain some errors, then the model outcomes will also be prejudiced or erroneous. Additionally, data management is challenging when dealing with large volumes of information that most organizations fail to address adequately.
How to Avoid This:
- Implement Data Governance: Implement stringent policies and protocols for handling data in the organization to improve data quality and standardization. This involves cleaning, normalization, and validation of the data collected and stored in the database.
- Invest in Data Management Tools: Employ sophisticated techniques and mechanisms for dealing with data overheads. They can aid in the collection, management, and retrieval of information in a systematic manner.
- Regular Audits and Monitoring: Schedule an audit regularly to ensure that data quality is fixed if it is of poor quality. This practice also helps in ensuring that the data collected is recent and reliable throughout the monitoring process.
It also has been identified that the quality of data and its handling directly affect AI models to provide better outcomes.
4. Ethical Concerns and Bias in AI Models
Ethical issues are another reason against AI usage: about 23% of companies mention it. Bias in implementing AI may also cause the software to be unfair or discriminatory to the users of the system and this is unforgivable as the reputation of the company will be at stake.
For example, if an AI system that selects employees for hire was trained on datasets that contained biases such that it would favor some groups over others, this would create biased hiring. This may lead to substantial ethical and legal issues that affect the business operations of an organization.
How to Avoid This:
- Develop Ethical Guidelines: Set ethical standards for AI utilization throughout the company. They should also cover areas such as bias, balance, and impartiality of the news.
- Bias Detection and Mitigation: Put measures to address possible bias in AI models. Ensure that the data used in the training of AI models is diverse and includes samples from different populations and test the AI models for biases at regular intervals.
- Ethical Audits: Schedule and perform ethical checks or reviews periodically on the AI systems to make sure they are ethical. This aids in preventing ethical issues from arising or at least helps in managing them before they get out of hand.
5. Integration Challenges with Existing Systems
IT systems currently in place may not support some of the new AI technologies hence leading to compatibility challenges. Such challenges include data silos, organizational and system legacy, as well as incompatible software. For instance, an enterprise that seeks to implement AI-based customer support services may discover that the chosen solution cannot easily interface with an old CRM system, leading to development challenges and higher costs.
How to Avoid This:
- Phased Approach to Integration: Reduce the complexity of AI initiatives by starting with less challenging projects that can be implemented without extensive system change. Build momentum as initial projects materialize.
- Use Middleware and APIs: Make use of Middleware and APIs when interfacing the implemented AI solutions with other systems. This can be of immense benefit to an organization or business as it can provide a way of addressing compatibility issues to enhance integration.
- Comprehensive Planning: It is also crucial to develop an integration plan with specific timelines, available resources, and some possible threats. Tackle both the AI and IT departments because you need both for implementing an integration.
Final Note
Despite enormous opportunities for generative AI for enterprises, several issues can lead to project failures. These are followed by the absence of goal and application definitions, inadequate AI personnel and knowledge, data quality and control problems, moral questions and prejudice, and compatibility problems with existing frameworks.
That, however, is something that companies have to address as they move forward and as AI starts becoming mainstream. To ensure that the power of AI can be fully realized, enterprises should consult with AI experts who have built the systems they require. Companies like us help enterprises identify AI opportunities and implement generative AI solutions the right way.
Latest Tech Insights!
Join our newsletter for the latest updates, tips, and trends.