5 Common Mistakes to Avoid While Jumpstarting the Gen AI Journey
Sector: Data Analytics and Artificial Intelligence
Author: Nisarg Mehta
Date Published: 07/09/2024
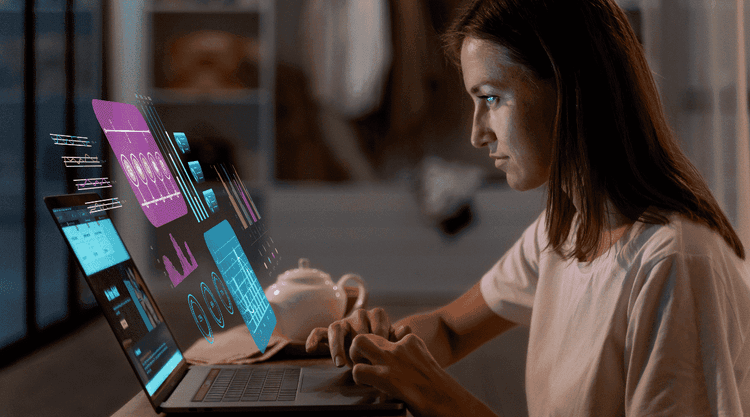
Contents
Generative AI (Gen AI) has quickly evolved from an industry-specific idea to being a tool that companies in different industries cannot survive without. It is surprising to note that as per Gartner, more than 80% of today’s enterprises will incorporate generative AI into their systems by 2026, which is evidence of the increased need for these systems. This growth is fueled by the capability of Gen AI that produces direct human-original text and visual, even source codes, for how businesses run and compete.
However, as with any digital transformation journey, the process of effectively establishing Gen AI is not without its share of hurdles. Therefore, recognizing and staying clear of pitfalls is imperative if this technology is to be utilized to the fullest extent.
5 Common Mistakes to Avoid While Implementing Generative AI
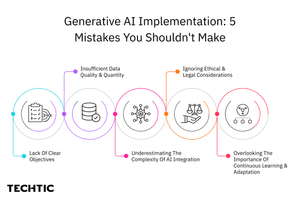
1. Lack of Clear Objectives
The most evident failure organizations commit when adopting Gen AI is the lack of specific goals and objectives. The lack of objectives provides a hazy direction, confusing the process and untimely usage of resources.
Importance of Clear Objectives
Businesses need to understand that Gen AI is not a solution to all business problems that will automatically fix every issue within a business. It is a mistake to treat it as such because it can result in overwhelming expectations and ultimately, project failure. Businesses often rush into the implementation of AI solutions without considering goals and strategies, and this leads to isolated and inconsequential initiatives.
Example of Unclear Objectives and Their Outcomes
For example, the management may decide to adopt Gen AI to generally enhance customer relations without defining what this enhancement entails. This can result in the use of solutions that do not solve the challenges, such as subpar call management or low problem-solving rates. This creates an ineffective and inconsistent approach to AI and its use that cannot offer meaningful improvements.
Guidelines on the Smart Goal Setting for Gen AI Project
To overcome such challenges, one has to establish the SMART goals, which stand for Specific, Measurable, Achievable, Relevant, and Time-bound goals.
- Specific: Have clear objectives when working on your Gen AI project. Avoid generic objectives such as stating that you want to “enhance customer service” instead say that you want to “cut average call handling time by at least 20%.”
- Measurable: Make sure your objectives are measurable in some way. It is suitable to use call handling time, customer satisfaction scores, as well as issue resolution rates in this case.
- Achievable: Have achievable targets that can be met by the organization’s human resources. The danger of setting impractical goals is often that they only result in discontent and the project being left unfinished.
- Relevant: Ensure that your Gen AI strategies are compatible with your business objectives or corporate strategies. AI initiatives should align with strategic initiatives like being a boon to customer delight or improving organizational performance.
- Time-bound: Develop time frames with which you intend to accomplish your goals. This fosters constant attention towards the project’s goals and objectives especially when there are time constraints.
Specific objectives also make it easier for organizations to ensure that their Gen AI endeavors are strategy-aligned and heading in the right direction toward achieving their goals hence making the implementation easier.
2. Insufficient Data Quality and Quantity
One of the critical errors in working with Gen AI is ignoring or underestimating the data quality and quantity factor. The utility of Gen AI solutions is highly dependent on the data on which the corresponding models are trained. Low-quality or insufficient data can cause AI to be nonoptimal, perpetuate bias, and produce inaccurate results.
Why High-Quality, Ample Data is Valuable
Accuracy and objectivity of data are among the key components of the effective Gen AI models. It is like the clay that is used in sculpture; it does not matter how efficient the chisel is; if the material is poor, the result will be poor. In the case of Gen AI, the efficiency, credibility, and functionality of the resultant model are functions of the quality and quantum of data fed to the model for learning.
Some General Mistakes Concerning Data
The following pitfalls can undermine the quality of data used in AI projects:
- Biased Data: If the training data contains bias, then the AI model will tend to reproduce and even exaggerate such bias resulting in unfair decisions.
- Incomplete Data: Missing data can lead to AI models that are not robust and generalizable across different situations, thus they may not be very efficient in practice.
Measures to Enhance Data Quality and Quantity
To overcome the data issues, organizations must adopt stringent data management practices:
- Data Audits: Find and erase prejudices in data collection by performing data audits as often as possible.
- Data Augmentation: Ensure data augmentation to increase both the quantity and variability of scenarios that are seen by the AI models when training.
- Data Cleaning: Before applying the most suitable approaches for the analysis, ensure the datasets undergo methods of data pre-processing such as cleaning to eliminate wrong values, conflicting data, and undesired characters or variables.
3. Underestimating the Complexity of AI Integration
One should know that it is not easy at all to integrate Generative AI (Gen AI) into the existing systems in organizations, this process is challenging and people often fail to estimate it. This can cause problems such as compatibility problems, scalability issues, and downtimes.
AI Integration Challenges
One of the challenges of adopting Gen AI is the compatibility of the new AI features with the already developed systems. This process often demands changes to conventional work patterns and this can be invasive if the process is not well coordinated. This causes compatibility problems especially if the existing systems are unable to meet the features required in AI models.
Potential Integration Issues
- Compatibility Problems: The implementation of Gen AI generally involves extensive alterations in the present IT architecture. Integration with new advanced tools may pose a problem because legacy systems may not support new AI technologies.
- Scalability Issues: If Gen AI is to become widespread then the system will have to grow in capability to feed this increased need. Failure to consider scalability is, therefore, a major problem that can face organizations seeking to integrate AI.
AI Integration Tips for Introducing AI into Your Business Model Successfully
To ensure a smooth integration of Gen AI, organizations should adopt best practices that include phased implementation and thorough testing:
- Phased Implementation: It is recommended to begin with pilot implementations to introduce the integration to a more restricted environment. This is helpful in solving all the possible challenges that may be associated with the actual implementation of the system.
- Scale Smart: After the successful implementation of the pilot phase, plan to scale the Gen AI solutions to other aspects of your organization.
- Thorough Testing: Continue with thorough testing that will validate the compatibility of the implemented AI model with other systems. These are the compatibility tests, performance tests, and user acceptance tests.
4. Ignoring Ethical and Legal Considerations
Before beginning to implement Gen AI, one must take certain fundamentals like ethical and legal considerations in mind. Failure to consider these elements poses considerable threats that range from violation of privacy to achieving prejudiced results and failure to meet the legal requirements.
Ethical and Legal Concerns in Gen AI
Gen AI may affect different spheres of society and open up critical legal questions. Privacy is always a concern since AI systems in most cases involve the use of extensive data, some of which may be personal. If not well managed it can result in cases of violation of people’s privacy. Also, Gen AI can learn and mimic biases from the training data and bring in more unfair and discriminatory practices.
Examples of Ethical and Legal Pitfalls in Gen AI Projects
One weakness includes the fact that the decision-making process of AI is not easily understandable. For example, the hiring AI model could be trained on prejudiced data which makes the system select some groups of applicants over others. This can lead to selective employment of certain workers and legal consequences. Another example is AI-produced content, especially deep fakes, which are damaging both for the target and for the credibility of the digital content consumers.
Guidelines for Ethical AI
To navigate these challenges, organizations should follow best practices for ethical AI development and ensure compliance with legal standards:
- Transparency: Assure that executive decisions based on Artificial Intelligence are transparent and can be easily understood. This fosters trust and means one party is answerable to the other.
- Fairness: Prevent and address biases in technology models that may hinder fair decision-making. This can include not only various types of data gathering but also intense checks to ensure that an algorithm is fair.
- Privacy: There must be strict governance policies in data protection to ensure that sensitive information is well protected. This includes masking data and making it unreachable to unauthorized persons.
- Compliance: This means that there should be constant updates on regulatory laws and policies that govern the use of AI and make sure that all applications are adhering to the law. This might include GDPR, HIPAA, or any regulations that are peculiar to the industry.
Focusing on ethical aspects and legal requirements will help to avoid commonplace mistakes and create reliable implements of Gen AI that do not violate user rights to privacy and openness while remaining fair at the same time.
5. Overlooking the Importance of Continuous Learning and Adaptation
One of the biggest errors that many companies make is that they see AI projects as a one-time investment where such a project will remain as it is once it is set up. This neglect can result in uncompetitive models, lack of optimization, and lost business prospects.
Gen AI models require constant development, modifications, and training to work as intended consistently. AI models are trained from data and can evolve based on the information provided, unlike traditional software. This constant training makes it possible for the models to remain effective and responsive to new and diverse scenarios.
Risks of Not Updating AI Model Data Regularly
- Performance Degradation: A model that is good today may be less so tomorrow if the environment and/or data change. This leads to performance degradation and results in low effectiveness triggering a lack of confidence in AI systems.
- Bias Accumulation: As is the case with training data, AI models, if not updated frequently, act as vehicles for reinforcing existing bias. Such biases are an issue that requires constant supervision and frequent training to be addressed properly.
- Technological Obsolescence: AI evolves, and new techniques and improvements appear quite often; It is imperative to keep abreast with the ever-changing world to ensure that one is not left behind.
Methods for Continuous Improvement
To ensure that AI models remain effective and up-to-date, organizations should implement the following strategies:
- Regular Retraining: Some of the best practices are; Updating the AI models with new data in relation to older models to ensure higher accuracy of data collected. This assists the models to work efficiently, especially where there are changes in existing conditions so that they continue to offer top-notch performance.
- Performance Monitoring: By evaluating AI models’ results and analyzing them periodically, it is possible to identify potential declines or shifts in performance and determine the effectiveness of certain AI techniques. One way of doing this is to use the performance indicators and feedback to make necessary corrective changes.
- Incremental Updates: In the meantime, it is wise to implement many emergent updates as compared to fewer considerable updates. This approach minimizes large-scale disturbances and is more reasonable to progressive changes for the better throughout close intervals.
- Adaptive Learning: Introduce elements for model learning where the AI models can continue learning from new data and feedback. This approach is useful since it assists the management in enhancing the models’ capabilities of adapting to various environments.
Conclusion
Altogether, one can conclude that there are many obstacles one might encounter on the way to Generative AI’s successful implementation. Thus, businesses are to adopt best practices and learn about the modern trends in AI to provide maximal opportunities for Gen AI. For those who are willing to start their Gen AI journey, Techtic Solutions is here to support and guide, so that you do not fall into the mentioned pitfalls and get your AI Right.
Latest Tech Insights!
Join our newsletter for the latest updates, tips, and trends.